Go back
Foundations Of Global Genetic Optimization(1st Edition)
Authors:
Robert Schaefer

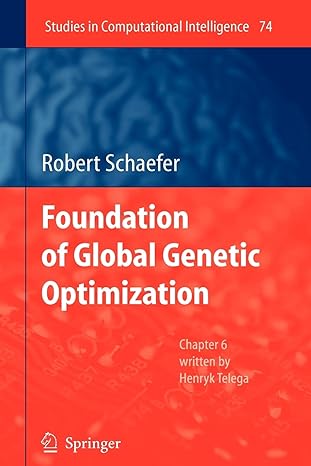
Cover Type:Hardcover
Condition:Used
In Stock
Include with your book
Free shipping: April 23, 2024Popular items with books
Access to 3 Million+ solutions
Free ✝
Ask 10 Questions from expert
200,000+ Expert answers
✝ 7 days-trial
Total Price:
$0
List Price: $80.70
Savings: $80.7(100%)
Book details
ISBN: 364209225X, 978-3642092251
Book publisher: Springer
Get your hands on the best-selling book Foundations Of Global Genetic Optimization 1st Edition for free. Feed your curiosity and let your imagination soar with the best stories coming out to you without hefty price tags. Browse SolutionInn to discover a treasure trove of fiction and non-fiction books where every page leads the reader to an undiscovered world. Start your literary adventure right away and also enjoy free shipping of these complimentary books to your door.
Foundations Of Global Genetic Optimization 1st Edition Summary: Genetic algorithms today constitute a family of e?ective global optimization methods used to solve di?cult real-life problems which arise in science and technology. Despite their computational complexity, they have the ability to explore huge data sets and allow us to study exceptionally problematic cases in which the objective functions are irregular and multimodal, and where information about the extrema location is unobtainable in other ways. Theybelongtotheclassofiterativestochasticoptimizationstrategiesthat, during each step, produce and evaluate the set of admissible points from the search domain, called the random sample or population. As opposed to the Monte Carlo strategies, in which the population is sampled according to the uniform probability distribution over the search domain, genetic algorithms modify the probability distribution at each step. Mechanisms which adopt sampling probability distribution are transposed from biology. They are based mainly on genetic code mutation and crossover, as well as on selection among living individuals. Such mechanisms have been testedbysolvingmultimodalproblemsinnature,whichiscon?rmedinpart- ular by the many species of animals and plants that are well ?tted to di?erent ecological niches. They direct the search process, making it more e?ective than a completely random one (search with a uniform sampling distribution). Moreover,well-tunedgenetic-basedoperationsdonotdecreasetheexploration ability of the whole admissible set, which is vital in the global optimization process. The features described above allow us to regard genetic algorithms as a new class of arti?cial intelligence methods which introduce heuristics, well tested in other ?elds, to the classical scheme of stochastic global search.
Customers also bought these books
Frequently Bought Together
Top Reviews for Books
Sara Mohammed
( 4 )
"Delivery was considerably fast, and the book I received was in a good condition."