Go back
Intelligent Data Engineering And Automated Learning IDEAL 2000 Data Mining Financial Engineering And Intelligent Agents(1st Edition)
Authors:
Kwong S. Leung, Lai Wan Chan, Helen Meng

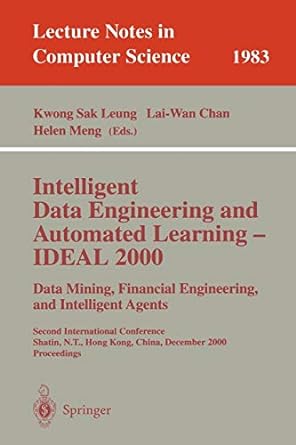
Cover Type:Hardcover
Condition:Used
In Stock
Include with your book
Free shipping: April 03, 2024Popular items with books
Access to 3 Million+ solutions
Free ✝
Ask 10 Questions from expert
200,000+ Expert answers
✝ 7 days-trial
Total Price:
$0
List Price: $6.99
Savings: $6.99(100%)
Book details
ISBN: 3540414509, 978-3540414506
Book publisher: Springer
Get your hands on the best-selling book Intelligent Data Engineering And Automated Learning IDEAL 2000 Data Mining Financial Engineering And Intelligent Agents 1st Edition for free. Feed your curiosity and let your imagination soar with the best stories coming out to you without hefty price tags. Browse SolutionInn to discover a treasure trove of fiction and non-fiction books where every page leads the reader to an undiscovered world. Start your literary adventure right away and also enjoy free shipping of these complimentary books to your door.
Intelligent Data Engineering And Automated Learning IDEAL 2000 Data Mining Financial Engineering And Intelligent Agents 1st Edition Summary: X Table of Contents Table of Contents XI XII Table of Contents Table of Contents XIII XIV Table of Contents Table of Contents XV XVI Table of Contents K.S. Leung, L.-W. Chan, and H. Meng (Eds.): IDEAL 2000, LNCS 1983, pp. 3›8, 2000. Springer-Verlag Berlin Heidelberg 2000 4 J. Sinkkonen and S. Kaski Clustering by Similarity in an Auxiliary Space 5 6 J. Sinkkonen and S. Kaski Clustering by Similarity in an Auxiliary Space 7 0.6 1.5 0.4 1 0.2 0.5 0 0 10 100 1000 10000 10 100 1000 Mutual information (bits) Mutual information (bits) 8 J. Sinkkonen and S. Kaski 20 10 0 0.1 0.3 0.5 0.7 Mutual information (mbits) Analyses on the Generalised Lotto-Type Competitive Learning Andrew Luk St B&P Neural Investments Pty Limited, Australia Abstract, In generalised lotto-type competitive learning algorithm more than one winner exist. The winners are divided into a number of tiers (or divisions), with each tier being rewarded differently. All the losers are penalised (which can be equally or differently). In order to study the various properties of the generalised lotto-type competitive learning, a set of equations, which governs its operations, is formulated. This is then used to analyse the stability and other dynamic properties of the generalised lotto-type competitive learning.
Customers also bought these books
Frequently Bought Together
Top Reviews for Books
Naje ali
( 4 )
"Delivery was considerably fast, and the book I received was in a good condition."