Go back
Perceptual Metrics For Image Database Navigation(1st Edition)
Authors:
Yossi Rubner ,Carlo Tomasi

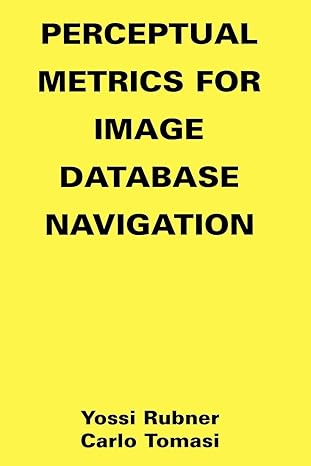
Cover Type:Hardcover
Condition:Used
In Stock
Include with your book
Free shipping: April 06, 2024Popular items with books
Access to 3 Million+ solutions
Free ✝
Ask 10 Questions from expert
200,000+ Expert answers
✝ 7 days-trial
Total Price:
$0
List Price: $162.93
Savings: $162.93(100%)
Book details
ISBN: 1441948635, 978-1441948632
Book publisher: Springer
Get your hands on the best-selling book Perceptual Metrics For Image Database Navigation 1st Edition for free. Feed your curiosity and let your imagination soar with the best stories coming out to you without hefty price tags. Browse SolutionInn to discover a treasure trove of fiction and non-fiction books where every page leads the reader to an undiscovered world. Start your literary adventure right away and also enjoy free shipping of these complimentary books to your door.
Perceptual Metrics For Image Database Navigation 1st Edition Summary: The increasing amount of information available in today's world raises the need to retrieve relevant data efficiently. Unlike text-based retrieval, where keywords are successfully used to index into documents, content-based image retrieval poses up front the fundamental questions how to extract useful image features and how to use them for intuitive retrieval. We present a novel approach to the problem of navigating through a collection of images for the purpose of image retrieval, which leads to a new paradigm for image database search. We summarize the appearance of images by distributions of color or texture features, and we define a metric between any two such distributions. This metric, which we call the "Earth Mover's Distance" (EMD), represents the least amount of work that is needed to rearrange the mass is one distribution in order to obtain the other. We show that the EMD matches perceptual dissimilarity better than other dissimilarity measures, and argue that it has many desirable properties for image retrieval. Using this metric, we employ Multi-Dimensional Scaling techniques to embed a group of images as points in a two- or three-dimensional Euclidean space so that their distances reflect image dissimilarities as well as possible. Such geometric embeddings exhibit the structure in the image set at hand, allowing the user to understand better the result of a database query and to refine the query in a perceptually intuitive way.
Customers also bought these books
Frequently Bought Together
Top Reviews for Books
Sheila Lucious
( 4 )
"Delivery was considerably fast, and the book I received was in a good condition."